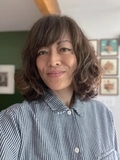
How to test AI: A practical guide for evaluating AI user experience and product design
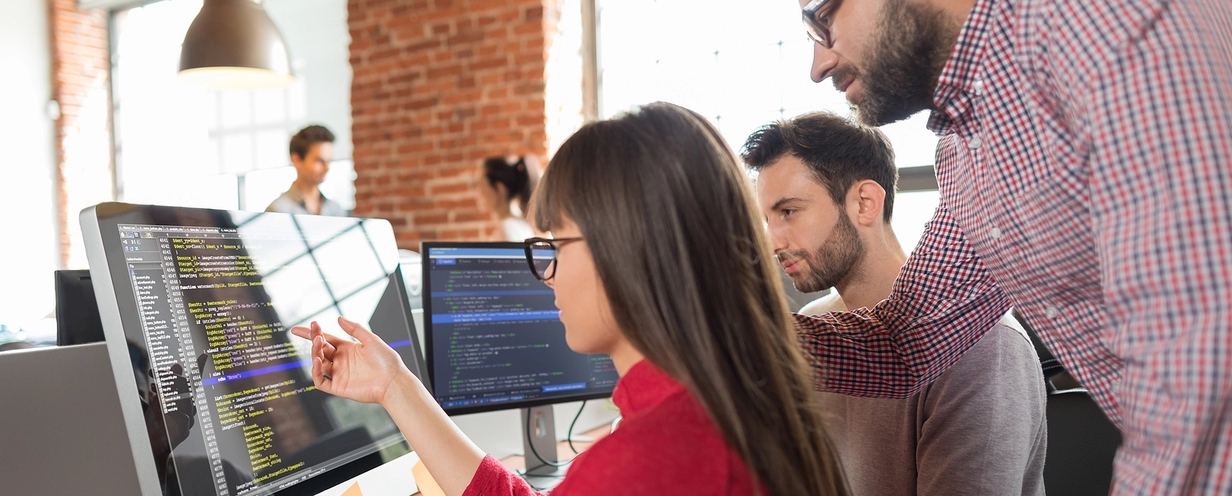
Anyone who has successfully shipped an app or a digital product can tell you that ideas, prototypes, and workflows undergo rigorous customer testing before they see the light of day. Successful product delivery teams understand how to embed UX research, especially AI UX research, into the product development lifecycle (PDLC) to de-risk decisions and help ensure a product-market fit.
What are ‘AI-enabled experiences’?
The definition of what qualifies as AI can get complicated. In this guide, we refer to "AI-enabled product experiences" as AI agents, copilots, backend AI systems, and digital interactions where AI plays a key role in the AI User Experience (AI UX) through personalization, recommendations, content generation, and more. We’ll refer to “AI” to capture a broad scope of consumer and business AI applications including machine learning and generative AI.
Common reasons AI experiments fail
A recent poll showed that only about half (54%) of AI proofs of concept make it into production. Understanding why many AI products fail is critical to safeguarding against potential pitfalls. Common reasons include inadequate problem framing, over-reliance on data without considering user context, and failure to iterate effectively on AI models and end user experiences. Learning from these failures can guide better AI UX research and development practices.
UPCOMING WEBINAR
Effective AI: how to choose the right generative AI features—and build them fast
In this webinar, you'll learn new AI best practices, including:
- How the conversational interface is changing the way people interact with technology
- How to optimize AI for emotional impact and credibility, not just usability
- How you can make AI functionality more discoverable, increasing user adoption
How testing AI-enabled experiences differs
Context setting
The integration of AI fundamentally changes some of the ways we traditionally build and test products. AI products and the markets they serve are dynamic. Our mental models around what an “AI” is can vary quite a bit. During a test, participants are being asked to evaluate something in an emerging market that they may have little or no frame of context around. In these cases, it’s helpful to anchor your conversation to AI experiences that are already familiar to the participant (such as ChatGPT) to enhance AI UX understanding.
The dynamic nature of AI models
Testing AI products involves evaluating the AI model's performance as well as the user experience. This includes assessing the accuracy and reliability of AI outputs, which can change over time due to data shifts and model updates made by your team or by 3rd party model providers. Unlike traditional products, AI products require evaluating both the experience and the model’s performance.
Recruiting for AI literacy, evaluating trust
AI is a nascent category full of unknowns. Those of us building with AI are figuring out how AI and humans should best interact, while consumers and businesses decide how they feel about AI. You’ll want to evaluate how user trust in AI and preferences on how AI shows up in their workflows shift over time. Consider segmenting your findings between cohorts like innovators, early adopters, and early majority. You can also screen participants for their understanding of AI as it can vary wildly from one person to the next.
Research stimuli and data
Complexities associated with AI call for extra considerations when preparing the research input. You may need a robust infrastructure for hosting and testing AI models with data that’s uniquely relevant to your participants to simulate a realistic AI UX experience, or host the model locally and have it process sample data. AI usability testing will likely require you to test against a higher fidelity prototype and add an evaluation of the system, its workflows, and how AI appears in the UI.
Methodologies and approach
In AI UX research, what participants say can often differ from what they do. The delta between the two can be more pronounced with AI. Evaluate verbal or written feedback in addition to behavioral data and reconcile the two to get a stronger signal for customer needs. Since most of us don’t have the historical data we need to estimate customer preferences around AI, consider increasing your testing frequency and leverage mixed methodologies across each stage of your product development. Alpha and beta testing will play more critical roles in setting your team up for success—as will post-production feedback collection—to help your team refine and optimize model parameters.
GUIDE
Effective AI: how to choose the right generative AI features—and build them fast
Discover how to build effective generative AI features that truly meet user needs—read the guide for 10 proven strategies, real-world success stories, and expert tips to get it right from the start.
Feedback implementation
Research readouts and recommendations will likely look different, as will the decision-making criteria and how teams iterate on the product. Research needs to inform not only product experience but how PMs and engineers optimize model parameters or conduct side-by-side tests of 3rd party model performance. Feedback also needs to inform sales and marketing activities as go-to-market teams optimize messaging to promote your AI products. It will be important to understand how various teams need to process the research feedback before a study is launched.
Legal and security requirements
Legal and permission considerations will also play a more significant role in the testing process, necessitating thorough clearance processes. You may need your participants to explicitly opt into the study or get their employer’s approval to engage with products that process their data, as many organizations have strict guidelines on how employees can engage with AI-enabled products. AI research comes with its own challenges, including data risks, user acceptance of AI, and the need to evaluate both expressed and behavioral data. Preparing for these challenges ensures a smoother research process and more reliable outcomes.
How testing AI-enabled experiences remains the same
Customer focus
Discovery is still about uncovering customer key pain points and unmet needs, not about integrating AI into your product for the sake of AI. At its core, AI and machine learning are technologies, a means to an end that enable us to more effectively solve problems. It’s critical to define the business requirements and why AI is being used to deliver unique value to your audiences.
What remains the same is the need to stay hyper-focused on customer problems and jobs to be done. A successful AI product addresses user pain points effectively, ensuring usability and satisfaction. An ideal AI experience seamlessly integrates AI capabilities to enhance user interactions. Key considerations include building trust and transparency and maintaining data privacy. Continuously evaluating the AI model’s performance and its impact on the user experience will be critical to your success.
Stakeholder alignment and expectation management
AI research requires a thoughtful approach to risk framing and expectation setting. Establishing clear goals, requirements, and testing parameters from the outset ensures aligned objectives across research, design, product management, and engineering teams. Effective AI product development necessitates close collaboration among all stakeholders. They will likely require more frequent testing and customer validation to de-risk AI initiatives, which tend to be costly investments. Decision-making processes around AI must be transparent and inclusive, ensuring all teams are aligned on goals and methodologies. Take this opportunity to build a deeper understanding of critical business decisions the team will be making, what’s at stake, and how research findings mitigate the cost of making uninformed decisions.
Final thoughts
With the appropriate planning, communication, and understanding of how AI products differ from traditional digital products, your team will set itself up to deliver experiences that resonate with your customers at the first launch. In this series, we’ll delve deeper into building your AI research plan, tips on audience recruitment, and an AI research checklist. Stay tuned for future installments where we will explore each of these topics in detail, providing you with the tools and insights needed to navigate the complex landscape of AI product research.
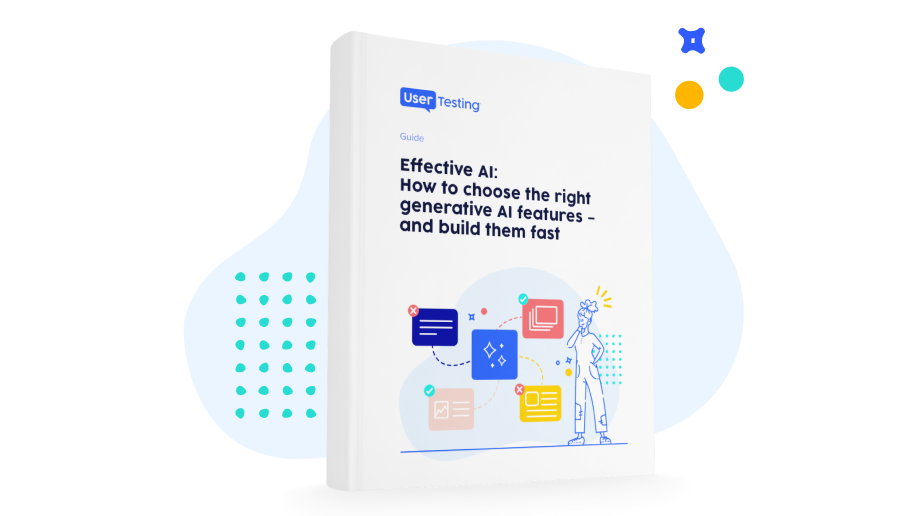
How to choose the right AI features
Learn how to choose the right generative AI features for your product—and develop them quickly.