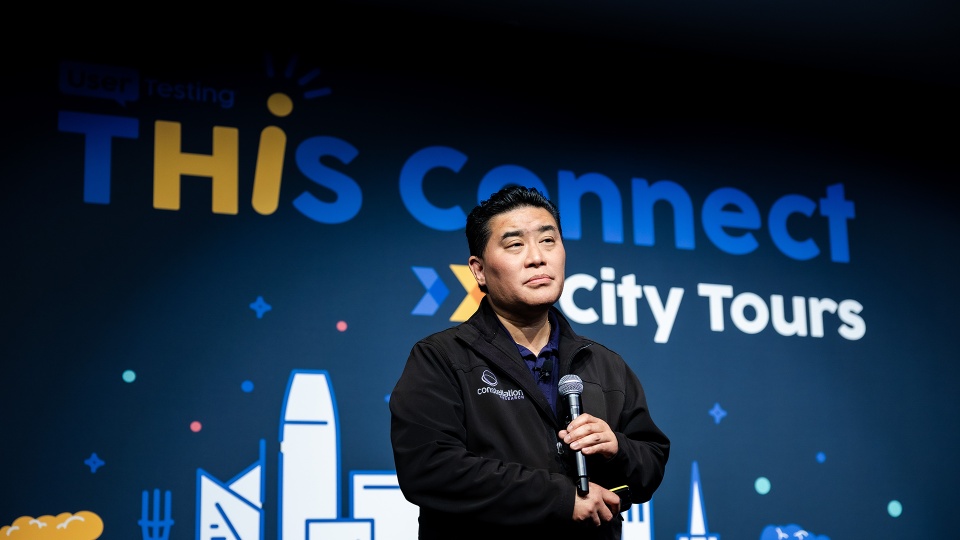
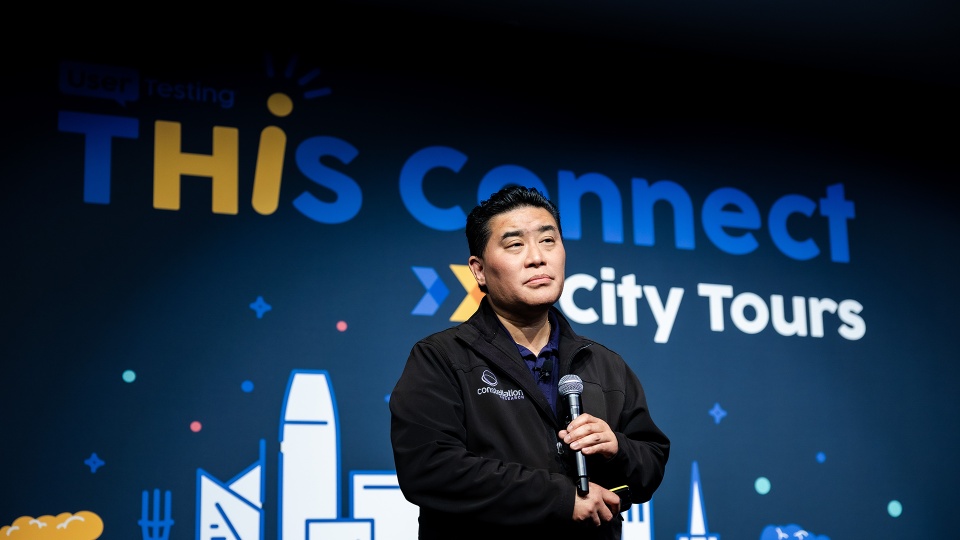
R “Ray” Wang: Mass Personalization at Scale for CX in an Age of AI
R “Ray” Wang
Principal Analyst, Constellation Research
AI powered experiences now provide the foundation for elevated CX. Learn how the end game of AI is better decisions and how leading teams are adapting, designing, and scaling AI. See how CX use cases come to life with AI and learn when and where to insert a human.
So up next, I am super, super excited to welcome this next guest. I've actually known this guy a long time. That's what happens when you're in technology a long time. You start seeing the same old faces. He doesn't need much of an introduction, so I'm just gonna say Ray Wang.
Alright. Ray Wong is with us. He's our tech guy. We wanna bring in Constellation Research principal analyst and founder, Ray Wong.
Ray, it's great to see you here. So lots to unpack. You got We can bring in Ray Wong, founder of the tech advisory firm, Constellation...
So up next, I am super, super excited to welcome this next guest. I've actually known this guy a long time. That's what happens when you're in technology a long time. You start seeing the same old faces. He doesn't need much of an introduction, so I'm just gonna say Ray Wang.
Alright. Ray Wong is with us. He's our tech guy. We wanna bring in Constellation Research principal analyst and founder, Ray Wong.
Ray, it's great to see you here. So lots to unpack. You got We can bring in Ray Wong, founder of the tech advisory firm, Constellation Research. Ray Wong is with us.
Everybody, good afternoon. How are you guys doing? Good. Alright. We're gonna talk a little bit about what's going on with AI personalization.
As everybody knows, this is everything from creativity to building decisions to taking it there. Also talk a little bit about the trends that are actually happening in terms of what that means in terms of our world. Think about the way that we look at research, the way we make decisions, the way we come to conclusions, the human bias, and, of course, all those things combined helping us actually build better products.
So let's start with a little interesting history lesson. We talked about, AI and companies that build AI are going to win ten to one. This is your companion. This is your ability to get to scale. And so I wanna go back into the history lesson.
Way, way back, someone invented this machine here. It's gonna change the world. It's gonna change our experiences, the future of work? Is it gonna change health care? Is it gonna change, how we actually deliver things to people? Anybody know what this is? Anyone else?
Steam engine? Okay. Yeah. Getting close. Anyone else?
Still? Yes. It could look like a still.
Yes, it kinda does. Alright. Let me give you a hint here. This was a really cool technology. Get ready.
Artificial refrigeration.
Whew, right?
Yeah, it actually started back in the 1800s. They started making some progress in the 1920s, and pretty soon, like, these were, like, the winners in the marketplace.
Anybody remember any of these companies?
Yes? Okay, some of you do. So who was the winner here? Yell out the winner, that came out, today's winner in the world of artificial refrigeration. Anybody?
Carrier. Okay. GE. Alright. Fair enough. Ready? Three, two, one. Why? Because they put it to work.
Right? They built the cold food supply chain, and in fact, their stock performance is better than anything else. And so I had the opportunity to speak in front of seventeen thousand employees at GE Appliances, and I actually put this slide up, and it was actually kinda funny.
And this was after their CEO said something really interesting. He said, everybody in this room will be using AI by the end of the year, and that's in marketing where we're building creatives and doing global campaigns. That's on the factory floor where we're looking at quality and manufacturing, and that's the point. Right?
We're all enamored by a technology, but in fact, it's really up to us to use it. And the people that are gonna be successful in AI are the ones that are going to be using it. Right? It's an augmentation.
It's our ability to actually expand what we do, and that's really where we are. And so we think first movers who actually use AI, whether they're using it to build better products, create better experiences, craft interesting journeys, that's what's gonna happen. Now this investment is coming. Right?
We see lots of things happening. There is AI arbitrage that's going on.
My son is a senior in college. He's a CS major, and he's about to join the ranks of unemployed CS majors.
Right? Because coding is gonna be automated. But he's got a lot of friends that are using all this code gen to do something else. Right? And they're gonna create very new types of work, and I think for him, that's gonna be an interesting place. So we think consumers will all have their own digital agents. We'll all have our own digital agents in place helping us do things.
How many of you guys, you know, start your first draft in some kind of AI tool?
You can admit it. It's okay. It's kinda common today. Alright. We got about a third of the room doing that, and that's why we think every employee, every individual is going to be able to do that.
So where are we in this? Well, couple years back, everybody said, oh, let's go do this. This is gonna be great. And then everybody jumped in.
People created pilots. They got past the pilots, and they started putting it into work, and they realized something was happening.
They don't have enough data to get to a level of confidence that they're going to trust. Right? So some things, you know, they're a little bit of a mistake. So if you're eighty five percent accurate, it's not too bad, you can still fill in the gaps.
But eighty five percent accurate, it depends on what you're working on. Eighty five percent accuracy in customer experience, not bad. Something gets routed to the wrong place, you can kind of fix that, nobody gets hurt. Eighty five percent accuracy in supply chain, oh, that's bad.
You're going to lose a million dollars a minute. What about eighty five percent accuracy in finance?
Anybody wanna go to jail?
What about eighty five percent accuracy in health care?
Oh, yeah. That's not good. So over time, what we're doing is we're adding other models. Right?
We're getting adding small language models where that gets us to the ninety five percent. And in some place, we get to very small language models. We'll get to a hundred percent. This gets to the point, there's there's lots of AI.
There's generative AI. There's agentic AI. There's classic AI. Lots of different approaches here. The main point is a little bit different.
The main point is what we have to do is figure out what is the right tool for what we're trying to do. If it's creative and you're okay with one plus one equaling two ninety nine point three percent of the time, not bad. If you actually have to make sure it's always that, you're gonna use a different tool. And so we're in this world where we've got generative AI helping us become creative, expand patterns to agents that are helping us do work, the classic ML and AI of figuring out hard types of problems.
All these tools are gonna be used at the same time. And when you think about that in the notion of testing, right, you're gonna try different tools for different approaches. Right? What you do for product testing in the drug world is gonna be different for product testing in a marketing campaign versus what you're gonna do for a customer experience journey mapping for a retail fraud.
And that's where we are. Now the broader trends in this marketplace are a little bit different. We are facing some interesting big shifts. There's massive margin compression.
We're squeezing margin out of everything we can. People are working as hard as they can to get stuff in place. We're also trying to grow. People are trying to find that next flywheel, the next subscription, that next service, the next membership, whatever's going to make us move there. And we're also in the midst of trying to figure out how we actually take these experiences and differentiate.
All the products look the same. The services look the same. How are those experiences? What are those outcomes? What gives you that emotional reach and connection?
And how do we test that? How do we know that works with the right set of personas? And, of course, we're also doing that and putting in the front lines so that if you do have front line employees, what can you do differently?
We were at a conference, in September last year where we're interviewing the CEO of Sam's Club, and we're asking, how are you using AI inside the organization? And this is a great example.
He said, we got rid of a hundred million tasks. Now at Sam's Club owned by Walmart, we're like, oh, what did they do? They must have fired all these people. Right?
But he said something very interesting. He said we put three percent more workers on the front line to greet people because they're not doing things like counting packets or figuring out what's in place or fixing things that we were able to do before. They had automated those tasks so they put people in front to do the customer experience. And so you're seeing shifts like that all across the board.
But we're also in a world what we call exponential efficiency, and that means you have to be efficient at the scale of AI. It is machine scale we're looking at, which is very different. Let me walk you through this tenets rule that we're actually going through. So think about this.
Boeing versus, I don't know, SpaceX. Very, very interesting. Right?
So the rockets that Boeing's sending up is ten times more expensive than a rocket SpaceX is sending up, and that's a slight problem.
Take this for example. A drone in the Middle East that we have is about a million dollars. Actually, no. A missile that we have in the Middle East is about a million dollars, and a drone is about a thousand dollars. Think about this, payments.
How much do you pay for ACH or a wire transfer? Twenty five dollars? ACH a dollar fifty? In India and Brazil, you send money to each other for free.
It's zero cents. Here's another one. You could be spending a lot of money on software. You could be spending thirty dollars a month for everything here.
So this is what we're talking about exponential efficiency. All across the board, I'll throw it this way, if you're not ten times cheaper, ten times better today, you won't get to play. So it's a ten x factor today that we're working in the world of AI.
In the next three years, you have to be ten times better and ten times cheaper.
And in the next five years, you have to be ten times better, ten times cheaper, and ten times faster. And this is really where AI is coming. That's a thousand x that we're talking about. And so this shift that's happening right now is really what we're talking about.
That's the exponential efficiency that's happening. Someone brought up the example of Twitter earlier. That's a very interesting place. They got rid of two thirds of their engineers.
What did people say?
It's gonna fall apart. Actually, it didn't. Right? Because it's all automated at this moment. Right?
So the point here is actually that you have opportunities to get to scale either by doing a thousand x more work with the same people you have or shifting actually how you balance. And every company is gonna decide a different approach. But what you can do is a scale that you couldn't imagine before. It's not even linear.
It's geometric, and that's where we're headed. Now that actually applies to a lot of what we do in AI. It's how do we make faster decisions.
Joe reminded me this is also about creativity. How do we come up with more iterations?
Right? And I'm gonna focus on decisions a little bit here because we start by asking some business questions, like, do people like a versus b? We've done a b testing for years. Okay.
Great. Now imagine a to x testing. How would you do that? What's that level of scale?
You can run more tests than you could before. So the business question starts there. The second piece is how do we build feedback and signals back into the process? Right?
Ninety percent accuracy, not bad. Ninety five percent accuracy takes a lot more time. Ninety nine percent accuracy, even more time, more data. And how do we get to that scale?
Then from there, what we wanna do is continue to augment this and get the precision decisions. We're testing all the time. We're testing in the product. We're testing in the experience.
And at some point, you're in continuous testing mode, and you're making iterations on the fly. You're changing product design on the fly. And at some point, we're gonna get this this thing called decision velocity.
You and I make a decision very quickly, but it takes us how long to get out of management committee?
A month?
A quarter?
A year? Maybe never?
Machines have already made a thousand decisions per second, and that's really the scale we're talking about. So exponential efficiency is happening not just in your ability to be faster, better, and cheaper. It's also happening in our ability to actually make decisions more quickly, make iterations, make product decisions, make changes along the way. And it's happening at scale.
We do it because things are in the cloud. There's data. We ask the business questions, which are analytics. We automate the process because we can't capture everything manually.
That's coming through computer vision. And, of course, the last part, which is really using AI to get take us to that level. And so it really comes down to that knowledge, the graphs that's in there. You're capturing all the context.
What time was it? What facial expressions did they make? Where was their eye tracking? Where did they go?
All that information and insight is constantly being built and that's your competitive weapon. That's what gives you that source for inspiration. That gives you the ability to figure out where to innovate. That gives you where all the iterations are there.
And so we're at a point where we're going to build autonomous systems from where we are. We're taking the base of interactions that we have. We're overlaying some social and the engagement. We're building journeys in terms of the experiences.
And from those experiences, we're crafting those contextually relevant experiences so that at some point, we're gonna build autonomous systems. We're gonna hear a lot about autonomous systems in the future, but that's actually where these iterations are gonna come. The more you data you put in, the more you digitize, the more you can actually deliver on these AI capabilities.
And so some things are gonna happen. And every task, we're gonna figure out when do we automate and, actually, when do we use humans. So highly repetitive tasks, it's good for pattern recognition. It's great for training, but that's going to go to automation and AI.
If we got lots of volume, we can't handle that. We're humans. Right? So we have to find a way to scale up.
Massive nodes of interaction, yeah, you might be able to multitask three things, five things, but you can't multitask a thousand things. Right? And if you think about time to completion, you don't want to put more people there. You want to get to some level of skill.
But when it gets to complexity, this is where it gets interesting.
It's very hard to figure out a complicated equation or algorithm that's going to go to machine. But there's so many decisions that we make today that cannot be modeled by math, and that's where the human side comes into place. Also in creativity, we might get a jump start in creativity, get three or four ideas out there, but the rest of it's coming from us. And you're gonna see a lot of the creativity on the human side of the equation.
And then the last piece is sometimes you want a human touch, sometimes you don't because it might be dangerous, you can't be there, or it might be one of those things where it's so important that you have to have someone there to actually be in the process and that physical presence becomes important. Now the goal here is a little bit different. Our goal here with all this AI is to be able to get to some of these things called situation awareness. So we start out by saying, what's going on?
They look around, lots of information. They're like, oh, that's way too much information. Give me an alert. Give me a notification.
And then over time, you're like, oh, wait wait wait. If you can do that, make a recommendation, make a suggestion.
Right? Give me what it is or take the props that you give and provide those prompts to actually get to those recommendations. And over time, I'm gonna learn from you what you like, and I'm going to automate that. And then at some point, I can make some predictions. I can help you prevent a disaster from happening, and at some point, we deliver on situational awareness.
Now every part of the process that you're in, in the testing process, in the creativity process, or even the decisioning process, you have to ask these four percent.
Trading curbs kick in, everybody calms down, the algorithms are reset, and we start off and go over again. It already happens today. Where do we augment the machine with a human? Now that's an interesting question.
Let's take a different industry. Let's look at health care. If I told you my AI based physician is eighty one percent accurate, how many of you would come see my AI based physician versus your physician? So who says eighty eighty one percent accurate AI physician?
Raise your hands.
No takers?
Oh my god. Not a single taker. Alright. Fine. If I told you my eighty one percent accurate AI based physician gets smarter over time, your regular physician is not keeping up on their continuous medical education, and has access to everybody's records and learnings over time.
Who would take my eighty one percent accurate AI based physician? Me. Maybe. I got a maybe.
Let me tell you if the actual human physician accuracy is only seventy seven percent. Are you ready for my AI based physician? Yes. Now remember, my physician's gonna get smarter with time.
Your physician will age out.
Who's in on my AI based physician? Okay. So you get the idea. We're gonna augment the machine with a human because what we wanna avoid is false positives, false negatives.
We don't wanna get into situation where we make a mistake. Right? That's very important. Now we're also gonna get to a point where we augment the human with the machine so you can actually make faster decisions.
That's what's happening right now. You're getting these tools that allow you to augment things. Stability is helping you actually get more creative. Perplexity is helping you answer a question.
Right? Some people are cheating. There's some really cool apps for interviews. I'm sure you guys all saw those.
Those are very cool, actually. But you get the idea. Now at some point, we're gonna have to trust human judgment, and we go to the human.
Every process, we're gonna do that. But the design point has changed. The frame shift has changed. It's not about where you automate, it's about when and where do you insert a human.
Now this is a really important question.
Why do you hit pound zero or zero pound when you call contact center agent? Anybody?
Bypass.
Bypass. Right? Because the menu sucks. Takes too long. Right? I thought they used user testing for that.
Right? Okay. Anyways, just kidding.
Alright. Why else? What else? Why else do you hit zero pounds? Used to it. Mental model.
Used to it. Mental model. Talk to a human. Who else?
You can be fast. You can be what?
Your issue will be resolved faster. That's true. Okay. We're getting closer to the real answer.
We're talking to humans quicker. Talking to a human quicker. But why do you wanna talk to the humor? Human.
You don't think that the IVR will work. You don't think the IVR will work. What else?
The human will empathize. Who else? You are human. You are human. Human to human.
Okay. Let me give you the real reason.
You're really calling and talking to another human because you wanna argue with them to get an exception in your favor.
Is that correct?
I'm not a mentalist.
Right?
I'm counting on the other person to give me an exception.
A five dollar rebate. I screwed up on my bill. Can I not pay my late fee? Come on. Right?
Okay.
So at some point, it's gonna come out like this. Like, your customer lifetime value is so good, you get to talk to a human so I can lose a little money on you. Right? Think about that one. But if your customer lifetime value is not good enough, you won't get to talk to Hume like all consumer tech companies in the Bay Area.
Try getting a refund for any of these consumer tech companies. I can't even find someone. Right? Okay.
So you get the idea. So so the design point is when and where do you insert a human is going to change everything that we do. Now in the process, we go through the cycle every time. We learn what's happening.
We try to figure out what happened. We try to make a recommendation, we test it, we act on it, and we're like, what happened? And we try to refine it. It's happening at exponential efficiency.
That's happening a thousand times per second, right, with every interaction, every connection point. And so what we're doing right now is we're gone from augmentation, okay, this thing's kinda helping me, to some level of acceleration that's moving faster, to some level of automation, and now we've got agents and soon advisors that are gonna be doing that work.
Okay? It's a natural progression that's going to happen. If you're not using the AI, you're not going to get there. Right? That's part of your piece, your ability to learn how to use AI is part of it.
Now all this comes back to the conversations we had about digital, and this is where mass personalization comes at scale. You guys remember the choose your own adventure books? Right? You start on page one, end on page three, come to thirty one, make a decision, you die, you flip back and start over again.
Right? That's what we're doing at scale. It's a massive choose your own adventure book. So let me walk you through an example.
This is a toaster. It is a forty dollar toaster. Right? Why are we looking at a forty dollar toaster?
You'll find out in a few minutes.
Who fills out the warranty card for a forty dollar toaster? Raise your hands.
There's always one person. There's always one person. Anybody?
Alright. You can be brave if you do.
Now usually people fill out that warranty card. There's usually one person. There's none in this room, so it tells me a lot.
Because they're afraid something might happen. Right? It breaks. I wanna get my warranty fixed. I wanna look at it.
And what I try to do is convince people to fill out the warranty card, and it's just a disaster. But imagine if I said, plug this thing in. Just give me a phone number, an email. It could be a junk number.
Let me monitor the toaster, see how it's going. And I can tell you that when the toaster breaks or something's about to happen or if it's about to die in thirty days, I'll give you a warning. Who would sign up for that?
Right? Protect your privacy. We're not gonna sell your data. All that kind of stuff. Right?
Alright. Got more people now. Thanks.
We are in the US. Really, Canary Islands of privacy. So but okay. So now you're gonna do this.
Now what's happening when I'm monitoring this toaster? Some interesting data sets come back. I know what features are being used. I know why toasters in Denver outlast toasters in Houston.
Anybody know why?
Humidity. Humidity.
Why do toasters in New York break more than toasters in San Francisco and it's not the New Yorkers?
No. But what else? Say that again.
Bagels. Bagels.
You're trying to jam the bagel in there and it breaks.
Okay. So now I'm doing customer experience monitoring for free. I know what features are being used. I know what features aren't being used. I know what GEOs with the people like. I I know what New Yorkers do with their bagels. Yes.
And suddenly, I can actually use that and create an underwriting model because I can take that user data and actually create brand new business models, and that gets exciting.
Right? So for example, if I said, buy this toaster for buy this toaster, and what it'll do is we'll monitor the toaster. And if the toaster ever breaks, we'll send you a brand new toaster in forty eight hours or less. We'll give you this underwriting model built into the toaster.
Would that be exciting to you?
Yeah. Okay. It's a forty dollar toaster. Even better. I'm gonna actually do something different. I'm gonna offer you a seventy five cent per month, five year program for Toast as a service. Who's in?
This includes the monitoring and, of course, a free toaster if it breaks in forty eight hours. Alright. Fine. I'll send you a brand new one. And then pretty soon, I can actually start cross selling you different toasters. Now you're wondering why I bring this up to you because the data sets that you started with testing suddenly provides you ability to build a better feature, suddenly gives you the ability to build an underwriting model on performance and outcomes, and more importantly, allows me to create a subscription model on toasters. And now I can go direct to you with a toaster offer and actually sell you other things.
Now if I didn't sign you up for the warranty card, what would the customer experience be like on the first call from you to the manufacturer of the toaster?
It would be horrible.
Hi. I bought this toaster. Okay. Where'd you buy it? Oh, I bought it at Target. Okay. Great.
Can you tell me what the toaster looks like? Oh, okay. What model is that toaster? Oh, I'm sorry. We don't have that toaster. You called the wrong store.
Alright? You know nothing about the customer on the other end. But as soon as we digitized it, what do we do? We now know exactly the toaster, the model number.
We've streamlined the customer experience on the service call. We know you have this toaster. Actually, it's even better. We know your toaster is about to die in thirty days.
We're gonna offer you a brand new toaster.
Would you like to take the offer? For ten dollars more, we'll give you this toaster with another five year warranty. Now you actually create a whole bunch of business models. And what I'm trying to say here is the day that you start for just on the testing side builds everything out you need from a business model side for all these opportunities. Because now you can do customer experience monitoring, you can do remote field service, you can offer a subscription model, you can go direct to consumer, and now you can cross sell other things.
This is why it's so important to bring that process. And when you start building that testing piece, you get there. Now this is the secret to all mass personalization at scale for customers, partners, suppliers, and employees. And the reason I'm gonna give you a future of work example is because that'll be easier to kinda do this because you're all working on different types of products.
So imagine I walk into a fifty story building.
Right? I'm in the lobby. The computer vision says, oh, looks like the guy on the third floor. Oh, yeah.
The gait analysis shows like, hey. It's the guy on the third floor. There's, like, an eighty percent probability this guy is on the third floor. So I walk into the elevator, and it says, sir, would you like to go to the third floor?
Makes sense. Right? But I also have a meeting with my boss, and she's on the fiftieth floor. And so option two pops up and says, would you like to go to the fiftieth floor?
Pretty cool. Right? Looks at my calendar, says it's an opening. Fine. I've been trying to meet with her for three days, so now I got a meeting with her.
But then option three shows up and says, there are free donuts on the fifth floor.
Oh, which one do I take? Right. And so what have we just done here? Well, the first part of the equation is, let's say what's going on.
Right? So we're looking at the context. What's the time of day? Where am I? Okay.
About two in the afternoon. It knows the location that I'm in. It serves up content to say, what would you like to do? We collaborate and engage, and it serves up the information in the elevator kiosk.
Alright. And so personalization scale happens happens in place. So we serve up anticipatory analytics, which is the personalization at scale. And so now we gave you a couple choices.
We gave you three choices, and we gave you actions on the choices. So we now have a catalyst for action. So do I go to the third floor like I was planning to after lunch? Do I go visit my boss, which sounds like a great idea, or do I go get the donuts?
Anybody wanna take a guess?
I get the donuts and bring them to my boss. Okay. Good.
Now there's a value exchange that happens over here that's kind of fun. Sometimes it's money. We bought something. Sometimes it's nonmonetary.
Right? We had an action. I moved somewhere. Oh, now I've lost my slides. Oh, they're still there.
Okay. On the monitor, if you can pop them up. Whatever. And then sometimes we have consensus.
We scheduled a meeting or an appointment, and then we keep doing that over and over again, and that's what we do. That's mass personalization at scale. You can take any kind of you can take any type of in any type of customer experience journey, future of work journey, supplier journey, and then you'll be able to see that. And so the whole idea is to start with the digital footprint and the datasets that you have, and then what we wanna deliver is an immersive experience.
We do mass personalization at shale, and then what we do is we do a value exchange. And then from there, we figure out, does this happen all the time? Is that repetitive? Is there one time of opportunity?
Is it dependent on another action? And then from there, we then brew it all over again.
That's mass personalization at scale, and with AI, it gets even faster. Right? And so once you have your datasets in place, you can continuously do this on a testing loop, and that's how we build that out. Okay.
Now what happens? Well, our goal here is to make sure that we always think about trust and transparency in that dataset. We have to make sure the datasets are top notch. They're the right datasets.
That gets us to the next level. The second thing is we have to make sure that we understand that the datasets that we have from the signals have a half life. Sometimes that half life is shorter than others. And over time, what we wanna do is continuously replace that data because we need more and more signals.
Now the battle for this data means that we have to get there and get better data mastery. How do we build that into our place? And that's how we build more use cases. And we're gonna have new types of organizations that are built there, but the value of the data is in the context, is in the flat sides of the data, the time, the location.
I mean, what business process were you in? What part of the journey? Right? What was the humidity?
What was your heart rate? That's a very interesting question. Were you excited? I mean, if I could track serotonin levels, that'd be cool.
So what endorphins were kicked off? And so I wanna understand that. I wanna create these moments that matter across the journey. And so pretty soon, we're selling datasets, we're trading datasets, we're comparing datasets to build those signals.
As we get richer and richer pieces of data, then we can get to that next piece. Now here's the thing. Most organizations don't have enough data to actually get to that level. And so the trick, what we wanna do, start taking those small language models and build what we call our data collectives.
You wanna share data with your customer base to get there. Otherwise, we're gonna be in these dark ages of data.
Here's the truth. Most of the data has been scraped off the Internet. There's nothing new. Right? All you have are, don't don't kill me if you're one of these folks, you have Taylor Swift, you have NVIDIA SEO, and you basically have, like, Capital One ads. That's it.
Right? There's nothing else left.
Okay? All the real data is sitting inside your company. It's sitting within your partners, and it's about to be shared. And we think data collectives are important. Now I'll tell you a story that's different outside of here about data collectives, but it's not a true marketing example, but it shows you why marketing data and data inside organizations is so powerful.
So in the old days, when Boeing made real planes I love Boeing but when Boeing made real planes, they had this really great dataset which would tell you revenue per mile, cost per mile, profit per mile. They knew every route, like LA to Newark or San Francisco to London. They knew how much it cost to operate the planes, and they used that as a competitive exam competitive weapon, in all their deals against Airbus. And so what Airbus did was said, look. We'll work with all the airlines, all the engine manufacturers, and we're gonna share that data with you. And if you all participate in sharing the data, we're gonna use that data to make all the products better. And so we had the airline airline, the aircraft manufacturer, and the engine company all working together to share that data to improve operational efficiency and performance.
Imagine if you did that between the retail manufacturing distributor. Imagine if you did that in comms, media, entertainment, tech. Imagine if you did that across the hospitality value chain between the travel partners, the booker, and the actual entity.
That's how you actually make things better. And so we actually think in the future, it's gonna be a lot of data collectives because most of these projects will fail because we don't have enough data to get to a level of precision that your stakeholders will trust. And you're gonna see a lot more data sharing, but really for the purpose of improving customer experience, driving better efficiency. Of course, there's profitability and pricing dynamics, but that data sharing is gonna be very important across the board. Think about the set of data you have right now.
Right? That data is your competitive advantage, and you're gonna trade for it. We think winners are gonna merge, when they can pull that off. Now why are we doing all this?
That's a great question. Well, we're doing all this because there's a return somewhere. Right? And we look at seven areas, and we think about return on transformational, investment like this.
There's brand transformation. You're changing the perception of the brand. It's going to look very different. Right?
But there's also business model transformation as you think about where data plays a role. And there's also revenue and growth. How do we actually use this to drive more revenue, drive better, you know, more velocity in terms of purchasing, right, more clicks and advertising? And then, of course, there's cost effects.
There's cost reductions. There's regulatory compliance or operational efficiency that all play into this.
Make sure you have a good business case before you begin. This is one of the things that you start with the business problem, then you figure out how you wanna apply it. Otherwise, you're just using technology for technology's sake, and it doesn't work that way. It doesn't work very well.
Okay. So a couple of things. I'm gonna leave you a frame shift before we go to q and a. There are five things that you have to do differently in this age.
The first one is the most important one. When and where do you insert a human?
Always start there. Sometimes it's regulatory compliance. Sometimes it's empathy. Sometimes it's really for loyalty. Sometimes it's because exceptions need to be made and you need human judgment.
Sometimes that's just part of your brand. Right? Some brands are like, no humans. It's easy to go.
I'll give you a great example. Two in the morning, I check-in to a Fairfield Inn. I don't wanna see anybody. Do you wanna see anybody?
Well, I don't wanna see anybody. I want the remote key. I want everything ready. I wanna check out of there.
I don't wanna see a single person.
You come to my conference, which Joe has been, to the Ritz Carlton. You pull up. I want that person handing you champagne. I want the center going, pfft.
Smells like a Ritz. I want you walking to the lobby. I want you hearing the bagpipes. I want you the full experience.
Right? But it's about choice. But why didn't we insert a human is very important. The second one is something that we actually haven't figured out, is how do we operate machine scale with humans?
And that's when I said about the trading curves and the breaks. Right? We're humans. We can only absorb so much.
So we always have to build to human scale. But we have to understand that we're operating in machine scale, which is a very different thing. And so where you think about putting the brakes, where you make sure that the machines don't overwhelm the humans, where you make sure that humans actually work with machines properly, that's going to be very important going forward. Now the third piece is do you have enough data?
And the answer is no. The answer is always going to be no. So the question is, if you don't have enough data to get to that level of precision, who do we partner with? How do we build those data collectives?
How do we take those signals and bring them together and share them so that we can all benefit from that dataset.
And then the last piece, which is the most important Who do you sue when something goes wrong?
Now, why do I bring this up? Okay. So it's twenty fifty. I'm on an automated flight from San Francisco to New York.
Somewhere over New York, a bird strike happens. Sounds like a familiar story, doesn't it? Right? There's one hundred and fifty people on the plane, ten million people on the ground, and the computer is, like, trying to make a decision. Now for the computer and the AI, it's a really simple decision. It's hundred fifty people on the air and ten million people on the ground. You might kill a hundred thousand people down there, so the best possible action is to abort the plane.
But the good news is sometime in the two thousands, some guy named Captain Scully remember this guy? He lands a plane on the Hudson.
Right? And so there's a point zero zero seven percent chance that you could land the plane and everybody's gonna be okay.
What is the prompt that shows up when this happens?
Would you like to re up your insurance?
Pretty much.
Okay. So you see where we're going. Alright. So here's where we're at.
The world is changing super fast. We actually have a world where this AI is going to play a role to help us augment what we're doing. It is a world of exponential efficiency and we have to operate at ten X, a hundred X and a thousand X scale, but we can do it in a very smart way. We are all data driven.
And because we're all researchers and data driven in this model, we know that the data is to our advantage. And how we use that data to make chase choices, like when and where we insert a human, to make choices in terms of operating at human scale in a machine scale environment, to build mass personalization at scale by understanding what steps we wanna do and what choices we wanna give folks, that's gonna change the way our companies operate. And for every company that's there, you wanna start with who your brand is, what your ethos is, and then build the experiences from there. The good news, this is not a qualitative effort now.
The good news, this is quantitative, and you can actually take the data you have and all this testing and all the research and craft those experiences that you want. And more importantly, you can craft it in a way that everybody can get to the adventure that meets them. And if you do that right and you use AI to be the tool, you'll be one of the first companies to get there before everybody else. Thank you very much.