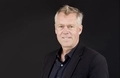
The history and future of UserTesting AI
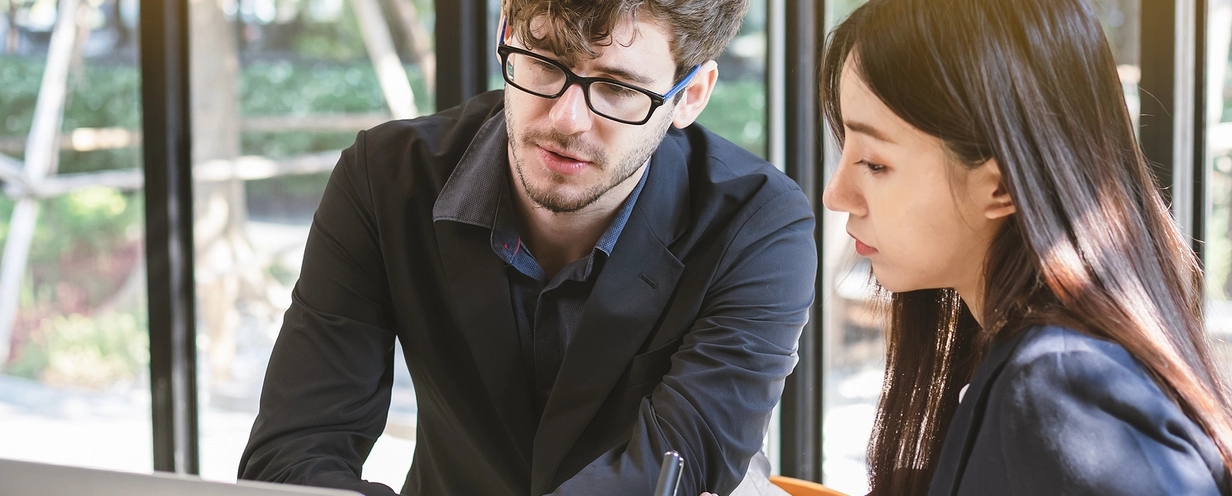
Last month, we launched the AI Insight Summary beta at the 2023 Human Insight Summit in Seattle, Washington. Our team spoke about AI’s potential and our vision for how it can empower experience research and foster a customer-first mindset for organizations across all industries.
The increasing popularity of large language models (LLMs) like ChatGPT has exposed the technology’s potential to the masses and interest in AI has never been higher. Of course, GPT is just one small example of the many exciting forms of AI and machine learning (ML) that are emerging. And the growing acceptance of AI and ML provides an exciting opportunity for our team to share some of the plans we have to deliver even more value to UserTesting customers in the future.
In this article, I’ll share our approach to AI, our AI history, and some of the upcoming advancements in experience research.
Approaches to machine learning
When it comes to machine learning in experience research, there are a number of ways to leverage generally available models and custom-built models based on your use case, resource availability, and in-house expertise. At UserTesting, we’re thoughtfully evaluating the optimal approach that will allow available models to work together to the best of their abilities.
Publicly available models
Generally available models like LLMs are trained on massive sets of publicly-available data. Because these models are learning from a vast amount of data, LLMs can understand almost any natural language input and generate an incredibly “human-like” response. This, however, is also the model’s weakness. Over time, the model’s outputs can become an average of all the information that’s currently out there.
There’s also the possibility of “model drift,” which is how the model’s behavior changes over time. As the model observes the most common types of prompts, it evolves to address common use cases and slowly shifts away from—and gets worse at—being able to answer outlier questions and domain-specific prompts. This phenomenon has been seen recently as it was discovered that GPT 3.5 and GPT 4 have become worse at solving math problems.
All of this to say: LLMs in the public model are incredibly useful for general, language-centric use cases. But they’re not always ideal for specialized environments that don’t center around language.
Proprietary UserTesting models
Specialized environments will be where SaaS organizations such as UserTesting shine. Because UserTesting has been entirely focused on experience research for the last 15 years, we have access to incredible amounts of data relevant to UX research. Access to this unique dataset, coupled with our domain knowledge and ML expertise, gives us a unique advantage in building and training bespoke models specific to experience research use cases.
Our unique collection of domain-relevant, proprietary data—which includes many forms of data like text, video, audio, and behavioral data—is perhaps our greatest differentiator. We have access to more than a decade of experience research data and a thorough understanding of customer needs, applications, and innovation opportunities. Not all of our ML R&D has resulted in wins. However, because of our early investment in ML, we have the necessary experience and learnings needed to leverage language-based inputs—and other data types—into models that generate reliable results for our customers.
You can easily imagine scenarios in which your employees’ experimention with emerging technology like GPT can lead to data risks. By embedding AI and ML into the UserTesting platform, we can enable customers to take advantage of emerging technology like GPT in a way that adheres to their security and compliance protocols.
Customer-built models
To solve unique business needs, many of our customers are also developing their own AI platforms and models using proprietary data generated by their internal systems. These are, of course, another vital piece of the experience research puzzle going forward. Custom models are an excellent solution for organizations with dedicated ML teams that have the expertise needed to train them and stay on top of emerging ML technology.
Leveraging the best of all models
The future AI landscape has room for all kinds of approaches. Organizations can use public models like LLMs for general-purpose needs, use UserTesting for specialized experience research use cases, and build models that are highly specific to their business. We see a great opportunity to ensure various types of models can coexist within an organization’s ecosystem and look forward to partnering with our customers to do so in the coming years.
A history of AI at UserTesting
While AI has just recently stepped into the public’s consciousness, UserTesting has been working with the technology for years. When I joined the company in early 2019, one of the first things I noticed was the large amounts of data we had that went largely unused. It became clear that even more data could be collected and used to help our customers speed up the analysis of their test results. The team knew that our unique access to domain-relevant data could allow us to create models that generated higher-confidence output for customers.
How the UserTesting data platform started
We started building out a data platform and populating it with data we already had, along with new behavioral data we started collecting. We built out a machine learning pipeline and hired our first ML engineer as an initial step in building out a larger ML team. We also acquired Truthlab, a machine learning startup with its own experience research data.
Over time, our team was able to successfully develop a patented data architecture that allows us to synthesize multiple types of data and understand the correlation between what participants are saying and what they’re doing. By converting behavioral input into natural language representations and producing behavioral transcripts, our models can look across various data types and identify spoken and unspoken insights.
Our first ML feature: sentiment analysis
Our first proprietary ML feature was the positive/negative sentiment analysis we launched in early 2020. We wanted to be strategically cautious when implementing ML into the product, so we initially called it “suggested sentiment” in order to encourage customers to maintain discretion when analyzing their data. We also made sure to build in a manual feedback loop from the start, so that customers can provide feedback on the analysis if needed—something we still consider a best practice for any ML feature.
Accelerating end-to-end research with AI
Since then, UserTesting customers have seen a steady stream of ML-powered features appearing in the product. The feedback on our ML features thus far has been resoundingly positive, with customers reporting much faster analysis timeframes. These features have begun to remove the analysis bottleneck, allowing for more testing, deeper insights, and—ultimately—more empathy across organizations. In addition to speeding up analysis, we’ve been applying AI to other areas of our product, such as automating quality control of think-out-loud sessions and improving test distribution to our participants.
Insight customization
Last year, we took the feedback loop introduced with the first sentiment feature to a new level with insight customization, allowing customers to identify and customize interesting and relevant findings using their own common, corporate terminology. This new feedback loop can make predictions in an organization’s own language, based on just a few examples—something we’ll extend to all current and upcoming ML features.
UserTesting’s unique approach to AI
From the very beginning, we’ve worked on combining multiple data sources into our data platform and ML models. We’ve created the largest purpose-built dataset of its kind for experience research, allowing us to deliver higher confidence results to our customers. We use aggregated and anonymized data from several sources such as the think-out-loud tests in classic UserTesting.
What the user says gets transcribed and combined with what they’re doing. This information gets fed through our data pipeline and into our platform. We then annotate and enrich the data with sentiment and smart tags to label what the user says, detect their intent, and determine if they struggle to complete a task. We also holistically assess the quality of the feedback, to provide customers with another level of assurance.
Data visualization
Data doesn’t mean much if it can’t be communicated. Our goal is to not only use AI to generate results, but to deliver the findings in an easy-to-understand format that allows us to quickly interpret the information and make customer-centric decisions with confidence. We’ve even patented the data processing that allows us to generate our Interactive Path Flow (IPF).
Managing AI hallucinations
More recently, we’ve started using large language models to analyze and summarize data. Because LLMs are generative in nature, they’ll always return something. If the AI doesn’t actually know the answer, it will make one up and sound confident in doing so. This is referred to as “AI hallucination.”
This can, of course, create problems if LLMs are expected to “know” things—which they don’t.
Through our research into LLMs, we’ve seen that combining data streams as inputs into an LLM generates more accurate results and reduces the risk of hallucinations. We’re also exploring the hosting and iteration of our own LLMs for more domain-specific tasks such as writing test plans and recommending audiences.
Earning trust with high-confidence AI results
With the largest purpose-built dataset of its kind and trusted ML models trained on domain-specific data, our AI/ML implementation delivers reliable, high-confidence results. But we don’t just expect customers to take our word for it.
We take an evidence-backed approach, meaning AI-generated results like the IPFs and AI Insight Summary point to the source videos. Think-out-loud tests, which are the majority of feedback collected through our products, maintain a link back to the exact video and audio clips that contributed to a particular result. This allows customers to verify the model output and see the evidence behind the AI predictions. They can drill down for further analysis and provide the human oversight needed to trust the results and make decisions with confidence.
We also have feedback loops and insight customization in place for customers to keep improving quality and mold the outputs to fit their exact use cases and language.
Maintaining human-centricity
Even with all the AI that powers our product, we still remain fundamentally human. Our ultimate goal is to foster connections between human beings and help customers stay in touch with the human side of business. We use AI to improve those connections—not remove them.
We will continue to invest in AI/ML—whether or not it’s in the peak or trough of the hype cycle— because we know it delivers value to our customers. We take trust in our product very seriously. The UserTesting team and I look forward to continually innovating on our AI solutions to accelerate time to insights and enable customers to build experiences that their own customers love.
Guide