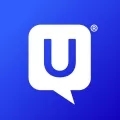
What are confidence intervals and why do we need them?
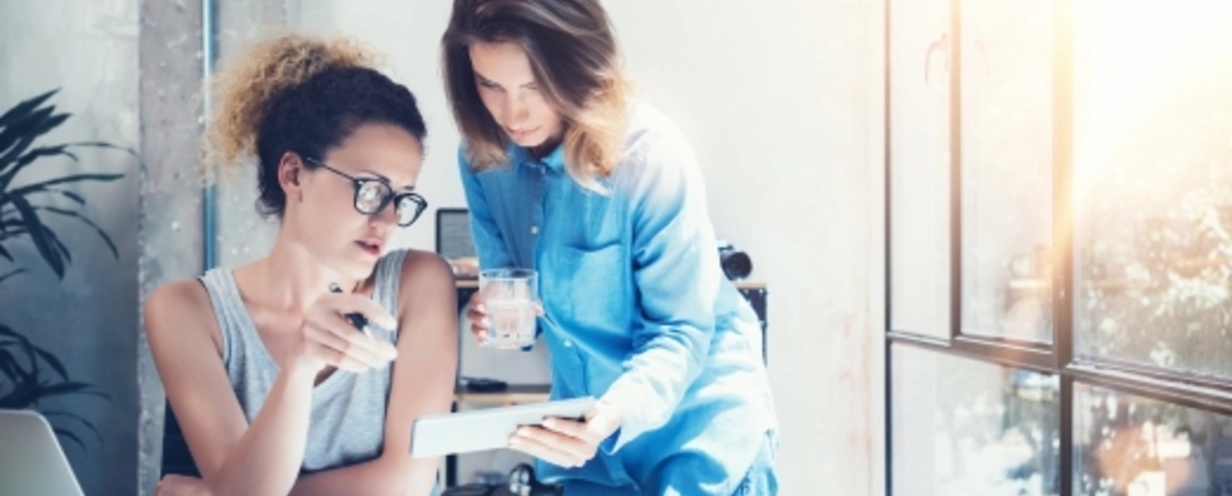
Why do we need confidence intervals?
When we run usability studies we are typically targeting a particular demographic, whether that is the general population, students in the UK, or women over 30 with at least one child as just a few examples.
Whatever our target audience, we can’t usually test all the people who are in this population due to the feasibility of access, time, and money.
Taking our UK student example, imagine we wanted to test two designs for a student bank account to see which design students preferred. In 2015-2016 there were 2.28 million students studying at UK Higher Education facilities. That would take a huge amount of time, cost and effort to ask all those students to rate how attractive they found the two designs. And that’s assuming we can get all of them to take part in our study.
If we did have access to all the students in the UK, and they all gave us their attractiveness ratings, the mean we could calculate for each design would be the actual population mean. The population mean is made up of scores from everyone who fits the demographic we want to test. It always exists, but is largely unknown, as we are very rarely able to test everyone in our population of interest.
What we are doing when we run our usability study, say using 100 students in the UK, is taking a sample of the population we are interested in.
This sample size is a lot more manageable and cost effective and we hope our 100 UK students will be representative of the population of all UK students.
From this sample data, we can get an idea of how well-received the designs are, and it allows us to make a more informed decision about which design to implement on the website.
The problem with taking samples is sometimes our sample mean will be similar to the population mean, and sometimes we might collect a sample and the mean is actually quite different from the population mean. This is due to something called sampling error.
We'd have no idea if our sample mean is a good or poor representation of the population mean. This where confidence intervals come in to help.
What are confidence intervals?
Confidence intervals are calculated from an estimate of how far away our sample mean is from the actual population mean. In other words, the term refers to the amount of error (or discrepancy) between our sample mean and the population mean. Confidence intervals provide us with an upper and lower limit around our sample mean, and within this interval we can then be confident we have captured the population mean.
The lower limit and upper limit around our sample mean tells us the range of values our true population mean is likely to lie within.
You typically see 95% confidence intervals reported but what does this mean? If we calculate a 95% confidence interval, we can be 95% confident that our interval contains the population mean. If we ran our study again, we would be confident our new sample mean would fall somewhere in this interval. If we calculate a 80% confidence interval we could be 80% confident that our interval contains the population mean and so on.
What does this percentage associated with a confidence interval represent? It is how sure, or confident, we want to be that our interval contains the true population mean. If we are only 95% confident our confidence interval contains the population mean, then 5% of the time we'll have a confidence interval that won’t contain the population mean.
However, we will never know whether the confidence interval we have obtained is one of those 5%. That's just something we have to come to terms with: 5% of all the confidence intervals you look at over your lifetime will not contain the population mean.
If we want to be highly confident our interval captures the population mean, we could calculate a 99% confidence interval. We would then be 99% confident that our population mean was captured within our confidence interval.
For example, looking at the same mean in Figure 1 below, we have calculated 95% and 99% confidence intervals, keeping the sample size and all other things constant. The 99% confidence interval has longer arms — in order to be more confident we have captured the population mean — so we need to increase the width of our confidence intervals.
Figure 1: 95% and 99% confidence intervals around the same mean with the same sample size and standard deviation
Why are confidence intervals useful in user research?
When we run studies, we want to be confident in the results from our sample. Confidence intervals show us the likely range of values of our population mean. When we calculate the mean, we just have one estimate of our metric. Confidence intervals give us richer data and show the likely values of the true population mean.
Sample size is one part of the equation used to calculate confidence intervals. If we increase our sample size (and kept everything else the same) we will see our confidence intervals reduce. When it comes to confidence intervals, the smaller the better. This is because we have a smaller range of values our population mean could lie within.
When time and money are tight in user research, sometimes we do have to rely on smaller sample sizes. However, by calculating the confidence intervals around any data we collect, we have additional information about the likely values we are trying to estimate.
Confidence intervals, although they may not seem it, are there to help. They make your data analyses richer and give you more from the metrics you captured while helping you to make more informed decisions about your research questions.

Watch a demo
See how easy it is to get fast feedback on a website, prototype, design, or more in this demo.